How AI-Powered Workflows Improve Faculty Performance Evaluation
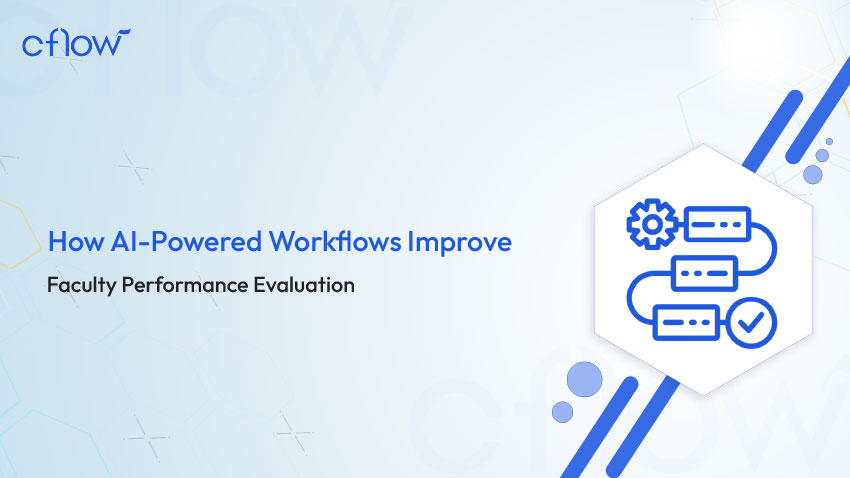
Key takeaways
- AI-powered faculty evaluation streamlines performance reviews, reducing manual workload and administrative inefficiencies.
- Faculty performance evaluation automation ensures data-driven insights, eliminating subjectivity in faculty assessments.
- AI in faculty assessment enhances fairness by analyzing multiple performance metrics objectively.
- Automated performance review for faculty accelerates evaluation cycles, ensuring timely feedback and performance tracking.
- AI-driven faculty evaluation systems improve decision-making by identifying trends, strengths, and areas for improvement.
- Workflow automation for faculty performance reduces administrative overhead and enhances collaboration among evaluators.
- AI for higher education performance management ensures compliance with institutional policies and accreditation standards.
- Smart faculty evaluation workflows enable real-time tracking, offering transparency and efficiency in faculty assessments.
Faculty performance evaluation is a crucial process in higher education institutions, ensuring that educators maintain high teaching standards and contribute effectively to student learning. However, traditional evaluation methods rely heavily on manual paperwork, subjective assessments, and inconsistent review cycles, leading to inefficiencies and potential biases.
With AI-powered faculty evaluation, institutions can automate performance assessments, providing data-driven insights, streamlining workflows, and ensuring fair evaluations. AI in faculty assessment integrates machine learning, workflow automation, and performance tracking, offering a smarter, more accurate, and transparent approach to faculty performance management.
This article explores how faculty performance evaluation automation enhances faculty assessments, improves accuracy, reduces biases, and increases efficiency in higher education institutions.
Table of Contents
Challenges of Traditional Faculty Performance Evaluation
Faculty performance evaluation plays a crucial role in ensuring the quality of education in higher institutions. However, traditional evaluation methods often rely on manual processes, subjective assessments, and inconsistent tracking systems. These inefficiencies make it difficult for institutions to provide accurate, fair, and timely faculty evaluations. Below are some of the most common challenges associated with traditional faculty performance assessments.
Time-Consuming and Labor-Intensive Process
Manual faculty evaluations require extensive paperwork, email exchanges, and in-person meetings, creating a heavy administrative burden. Academic administrators and faculty members spend countless hours compiling reports, documenting achievements, and manually entering data into spreadsheets or institutional databases.
The process is often repetitive, inefficient, and prone to errors, leading to unnecessary delays in finalizing evaluations. Without automation, institutions struggle to streamline review cycles, making it difficult to provide faculty members with timely feedback and performance insights.
Subjectivity and Lack of Standardization
One of the biggest drawbacks of traditional faculty evaluations is the lack of a standardized assessment framework. Evaluations often rely on subjective feedback from students, peers, and administrators, introducing biases that may impact faculty career progression.
Different departments may use varying criteria for faculty assessment, leading to inconsistencies across an institution. Without a structured, data-driven approach, faculty evaluations can become unreliable and unfair, making it difficult for institutions to implement objective and constructive performance reviews.
Difficulty in Tracking Long-Term Performance
Faculty members contribute to their institutions in many ways, including teaching, research, mentorship, and administrative responsibilities. However, tracking long-term performance using manual methods is challenging. Paper-based or disconnected digital records make it difficult to assess faculty growth over multiple semesters or years.
Without a centralized tracking system, institutions cannot effectively measure faculty contributions, identify trends in teaching effectiveness, or recognize achievements that warrant promotions or tenure. This lack of historical performance data makes long-term faculty development planning inefficient.
Inefficient Communication and Collaboration
Faculty performance evaluations involve multiple stakeholders, including department heads, deans, academic committees, and accreditation bodies. Traditional evaluation processes rely on emails, meetings, and paper-based forms, leading to communication bottlenecks and delays in approvals.
Faculty members often do not receive timely updates on their evaluations, while reviewers struggle to coordinate feedback effectively. The lack of a collaborative platform results in fragmented decision-making, slows down faculty assessments, and creates frustration among faculty members and administrators.
Limited Data-Driven Insights
Modern academic institutions generate vast amounts of performance-related data, but traditional faculty evaluations fail to leverage these insights effectively. Manual assessment methods do not provide real-time analytics on faculty performance, student engagement, or research impact.
Institutions relying on outdated evaluation processes miss opportunities to identify high-performing faculty, support struggling educators, and make data-backed improvements to faculty development programs. Without AI-driven analytics, faculty assessments remain reactive rather than proactive, preventing institutions from optimizing their workforce effectively.
Compliance and Accreditation Challenges
Higher education institutions must adhere to strict accreditation requirements and institutional policies when conducting faculty evaluations. Accreditation bodies often require comprehensive documentation of faculty contributions, performance reviews, and justifications for tenure or promotions.
Manual assessment processes increase the risk of missing documentation, failing to maintain proper audit trails, or struggling to provide compliance reports during accreditation reviews. Institutions that lack a structured and automated faculty evaluation system risk non-compliance, which can impact funding, institutional reputation, and faculty retention efforts.
By addressing these challenges, institutions can improve the efficiency, accuracy, and fairness of faculty performance evaluations. Transitioning to AI-powered faculty evaluation systems can eliminate manual inefficiencies, reduce biases, and provide real-time insights that help educators enhance their teaching and research contributions.
How AI-Powered Workflows Improve Faculty Performance Evaluation
Higher education institutions require an effective and transparent system for faculty performance evaluations. Traditional assessment methods are time-consuming, subjective, and prone to inconsistencies.
AI-powered workflows revolutionize the evaluation process by automating data collection, reducing administrative overhead, and ensuring compliance with institutional standards. With AI-driven faculty assessment, universities can ensure fairness, efficiency, and accuracy in tracking faculty contributions.
AI-Powered Data Collection and Analysis
AI-powered faculty evaluation systems enhance data collection by aggregating information from various sources, including student feedback, research publications, classroom engagement metrics, and administrative contributions. These systems use machine learning algorithms to analyze teaching effectiveness, student interactions, and faculty workload distribution in real-time.
Unlike traditional methods, AI eliminates human bias by identifying patterns and trends based on factual data. With AI-driven assessment models, faculty members receive comprehensive feedback that reflects their strengths and areas for improvement, allowing institutions to foster a data-driven culture of academic excellence.
Automated Performance Review and Workflow Management
Manual faculty performance reviews require excessive paperwork, slow review processes, and fragmented communication between evaluators. AI-powered workflow automation digitizes the entire review cycle, ensuring faculty evaluations follow a structured process. Performance data is automatically routed to department heads, academic deans, and accreditation bodies based on predefined workflows.
Faculty members receive timely feedback, allowing them to address performance gaps and improve teaching quality. Automated workflows also reduce human errors in documentation, ensuring every assessment is stored securely and can be retrieved for audit purposes.
Objective and Standardized Evaluations
One of the biggest challenges in faculty assessments is subjectivity. AI-powered evaluation systems address this issue by applying standardized metrics to assess faculty performance. Institutions can define specific parameters such as course completion rates, student engagement levels, and research contributions.
AI-based algorithms ensure that evaluations are consistent across departments, reducing bias and improving transparency in faculty reviews. With AI-driven faculty evaluation systems, universities can establish a fair and objective performance measurement framework.
Real-Time Performance Tracking and Analytics
AI-based faculty performance tracking provides institutions with real-time data insights through interactive dashboards. Academic administrators can monitor faculty performance trends and adjust professional development programs accordingly. These systems track key indicators such as teaching effectiveness, research output, peer collaboration, and faculty workload distribution.
Faculty members also benefit from self-assessment tools that help them measure their progress and adjust teaching methods based on student performance feedback. By leveraging real-time analytics, universities can proactively support faculty growth and optimize institutional effectiveness.
Enhanced Collaboration and Feedback Mechanisms
Effective faculty evaluation is not just about reviewing past performance but also about fostering continuous improvement. AI-powered platforms facilitate dynamic collaboration between faculty members, department chairs, and academic reviewers. Feedback mechanisms allow for structured peer reviews, student input, and administrative oversight.
Faculty members receive personalized recommendations based on their performance data, helping them enhance their teaching methodologies and research output. AI also enables automated notifications and reminders for faculty members to submit self-assessments or respond to student feedback, ensuring an ongoing performance enhancement cycle.
Compliance and Accreditation Readiness
Higher education institutions must adhere to strict accreditation requirements and institutional policies when conducting faculty evaluations. AI-powered faculty appraisal automation ensures compliance by maintaining audit trails, storing evaluation records securely, and generating compliance reports as needed.
AI-driven evaluation systems align faculty assessments with accreditation guidelines, reducing the risk of non-compliance during external audits. Institutions can use AI-powered documentation tools to track faculty contributions to curriculum development, research initiatives, and community engagement, strengthening their accreditation standing.
By integrating AI-powered workflows into faculty performance evaluations, higher education institutions can streamline assessment processes, enhance fairness, and improve faculty development programs. These technologies ensure that faculty performance evaluations are data-driven, transparent, and aligned with institutional goals.
Key Features of AI-Powered Faculty Evaluation Systems
AI-Driven Analytics and Performance Metrics
AI-powered faculty evaluation systems leverage advanced analytics to measure key performance indicators such as teaching effectiveness, student engagement, research productivity, and administrative contributions.
By analyzing vast datasets, these systems identify trends and patterns that help academic leaders make informed decisions about faculty development, promotions, and tenure eligibility. Institutions can track faculty performance across multiple semesters or years, ensuring a data-backed approach to continuous improvement.
Automated Evaluation Workflows
Manual evaluation processes often lead to delays, miscommunication, and inconsistent review cycles. AI-driven faculty evaluation platforms streamline these workflows by automating the entire assessment lifecycle.
From collecting student feedback to routing evaluations through different approval levels, AI ensures that every stage is efficient and error-free. Automated workflows reduce the administrative burden, allowing faculty members and review committees to focus on constructive performance discussions rather than paperwork.
Digital Faculty Evaluation Dashboards
Institutions often struggle with scattered faculty performance data across different systems. AI-powered faculty evaluation dashboards consolidate information in one centralized location, offering real-time visibility into faculty performance metrics. Faculty members can track their own progress, receive feedback, and access insights into their teaching impact.
Administrators and department heads benefit from instant reports and comparative analysis, helping them recognize high-performing faculty members and identify those who may need additional training or support.
Customizable Evaluation Criteria
Different institutions have unique academic policies, performance metrics, and accreditation requirements. AI-powered evaluation systems allow universities and colleges to customize assessment criteria, rubrics, and performance thresholds according to their institutional goals.
Whether evaluating teaching effectiveness, research contributions, or administrative responsibilities, AI-driven solutions offer flexible configurations that align with institutional and accreditation guidelines.
Secure Documentation and Compliance Tracking
Maintaining compliance with accreditation standards, institutional policies, and government regulations is a significant challenge for higher education institutions. AI-powered faculty evaluation systems provide secure digital documentation, automated compliance tracking, and audit-ready records.
Institutions can generate reports on faculty performance at any time, ensuring seamless accreditation reviews and regulatory compliance. Additionally, role-based access control ensures that only authorized personnel can access sensitive faculty evaluation data, enhancing security and data integrity.
By leveraging these features, higher education institutions can streamline faculty performance evaluations, ensure transparency, and drive continuous faculty development.
End-to-end workflow automation
Build fully-customizable, no code process workflows in a jiffy.
How Cflow Enhances AI-Powered Faculty Performance Evaluation
Cflow, a no-code workflow automation platform, enables higher education institutions to automate faculty performance evaluations with AI-driven workflows.
- AI-Powered Workflow Automation – Cflow eliminates manual inefficiencies, automating performance reviews, faculty assessments, and compliance tracking.
- Data-Driven Decision Making – With real-time analytics, faculty performance trends and insights are easily accessible for decision-makers.
- No-Code Customization – Institutions can configure evaluation workflows without coding expertise, ensuring flexibility in faculty assessment criteria.
- Seamless Integration – Cflow integrates with HR systems, student feedback portals, and compliance platforms, creating a centralized evaluation system.
By leveraging Cflow’s AI-driven workflow automation, institutions can ensure standardized, fair, and data-driven faculty evaluations, improving faculty development and institutional excellence.
Case Study – Analytical Approach for AI-Based Teacher Performance Evaluation
Background
Educational institutions have long faced challenges in evaluating teacher performance due to subjective assessments, inefficient manual processes, and inconsistent evaluation criteria. To address these concerns, researchers have explored AI-driven analytical approaches to enhance objectivity, efficiency, and accuracy in faculty performance evaluations.
This case study examines a recent study published in Springer that highlights an AI-based methodology for evaluating teacher performance using machine learning algorithms, data analytics, and automated evaluation systems.
Objective
The study aimed to develop and test an AI-based performance evaluation model that can objectively measure teacher effectiveness using quantifiable metrics. The goal was to move beyond traditional evaluation methods, which often rely on student feedback alone, and instead incorporate multi-source performance data, including classroom engagement levels, student outcomes, peer reviews, and research contributions.
Methodology
The research utilized a data-driven analytical approach, integrating machine learning algorithms and natural language processing (NLP) techniques to analyze faculty performance. The evaluation model was designed to:
- Collect real-time data from student feedback, faculty research publications, teaching engagement, and attendance records.
- Analyze trends using AI algorithms to detect patterns in faculty performance over multiple academic sessions.
- Apply predictive analytics to forecast potential areas for faculty improvement and professional development needs.
- Automate scoring mechanisms to provide unbiased performance ratings, reducing subjective bias from human evaluators.
The AI model was tested across multiple institutions and applied to diverse faculty groups to assess its effectiveness.
Key Findings
The results demonstrated significant improvements in the accuracy and efficiency of faculty evaluations compared to traditional assessment methods:
- Enhanced objectivity – AI-based scoring reduced evaluation bias by eliminating subjective influences from students, peers, and administrators.
- Faster evaluation cycles – Automated assessments cut down faculty review processing time by over 50%, enabling quicker feedback and decision-making.
- Data-driven insights – The AI model provided real-time performance tracking, helping institutions identify high-performing faculty and areas for improvement.
- Improved faculty engagement – Transparent and structured evaluation reports helped faculty members better understand their strengths and weaknesses, leading to improved teaching strategies.
Impact on Higher Education Institutions
The study highlights how AI-powered faculty evaluation systems can revolutionize the way academic institutions assess and develop their educators. By integrating AI-driven analytics, institutions can ensure:
- Fair, transparent, and unbiased faculty assessments.
- Efficient workflows that reduce administrative workload.
- Comprehensive performance insights that foster faculty development.
- Seamless compliance with accreditation and governance requirements.
Conclusion
The findings from this AI-based analytical approach underscore the transformative potential of AI in faculty performance evaluations. As institutions continue to embrace digital transformation, AI-driven evaluation models can provide unparalleled accuracy, fairness, and efficiency in faculty assessments, leading to overall improvements in higher education standards.
Final Thoughts
AI-powered faculty evaluation is transforming higher education performance management by introducing data-driven insights, automated workflows, and compliance readiness. With AI-driven automation, institutions can ensure timely, fair, and standardized faculty performance assessments, improving teaching quality and institutional governance.
Cflow offers a comprehensive, no-code faculty evaluation solution that helps universities and colleges automate faculty performance tracking, streamline evaluations, and maintain accreditation compliance.
Ready to revolutionize faculty evaluations? Explore Cflow today and experience AI-powered workflow automation for academic excellence!
FAQs
- How does AI improve faculty performance evaluations?
AI enhances faculty evaluations by automating data collection, analysis, and review processes, ensuring fair and objective assessments. - Can AI-driven faculty evaluation systems reduce administrative workload?
Yes, AI-powered automation eliminates manual paperwork, automates workflows, and accelerates approvals, reducing administrative burden. - How do AI-driven evaluations ensure fairness?
AI uses standardized metrics and machine learning algorithms to minimize biases and provide consistent faculty performance assessments. - Are AI-powered faculty evaluation systems customizable?
Yes, institutions can configure evaluation criteria, performance thresholds, and assessment rubrics based on academic policies. - How does Cflow support AI-driven faculty evaluations?
Cflow provides no-code automation for faculty performance tracking, integrating AI-powered workflows, real-time analytics, and compliance management. - Can AI-driven evaluation systems integrate with existing higher education platforms?
Yes, AI-powered faculty evaluation tools can seamlessly integrate with HR systems, student feedback portals, and accreditation platforms for a centralized evaluation process.
What should you do next?
Thanks for reading till the end. Here are 3 ways we can help you automate your business:

Do better workflow automation with Cflow
Create workflows with multiple steps, parallel reviewals. auto approvals, public forms, etc. to save time and cost.

Talk to a workflow expert
Get a 30-min. free consultation with our Workflow expert to optimize your daily tasks.

Get smarter with our workflow resources
Explore our workflow automation blogs, ebooks, and other resources to master workflow automation.
What would you like to do next?
Automate your workflows with our Cflow experts.